Translate this page into:
Introduction to artificial intelligence and machine learning into orthodontics: A review

*Corresponding author: Rony T. Kondody, Department of Orthodontics, Sri Rajiv Gandhi College of Dental Science and Hospital, Bengaluru, Karnataka, India. ronykondody55@gmail.com
-
Received: ,
Accepted: ,
How to cite this article: Kondody RT, Patil A, Devika G, Jose A, Kumar A, Nair S. Introduction to artificial intelligence and machine learning into orthodontics: A review. APOS Trends Orthod 2022;12:214-20.
Abstract
Over the past few years, artificial intelligence (AI) and machine learning (ML) have revolutionized different healthcare branches, including dentistry. AI in a wider aspect means computers that mimic or behave like human intelligence whereas ML forms a part of AI and enables machines to increase their capabilities by the process of self-adapting algorithms. AI models’ basic principles or fundamentals are purely based on artificial neural networks or convolutional neural networks. This review focuses on giving a comprehensive and detailed explanation about AI and ML technology and their wide range of applications in various sections of orthodontic practice.
Keywords
Artificial intelligence
Artificial neural networks
Machine learning
Orthodontic practice
INTRODUCTION
The field of orthodontics majorly focused on improving the quality of life by identification of various craniofacial malformations and occlusal malrelation, thereby preventing, intercepting, and correcting various abnormalities of craniofacial structures. In recent years, a new trend has emerged, where the main focus is given to facial esthetics.[1] Various treatment options available for orthodontists include interproximal slicing, extraction mechanism, expansion of dental arches, and distalization procedure.[2]
Over the past decade, newer technologies such as artificial intelligence (AI) and machine learning (ML) have revolutionized the world. AI works on the principle of mimicking the human brain and being able to do various task that needs human intelligence.[3] Whereas ML focused on designing and applying algorithms that can learn things from previous cases and this technique includes parameter adjustment with respect to underlying techniques such as, number of neurons, layers in a neural network technique; size of population, rate of mutation and crossing over rate in genetic algorithms etc.[4]
The subfield of ML is called deep learning (DL) refers to artificial neural networks (ANNs) with a complex multilayer system that consists of layers and interconnected neurons which help in visualizing minute details such as corners, lines, and edges that help clinicians optimize future decisions and improve the quality of care.[5] The term “Clinical decision support system (CDSS)” is a reference, particularly useful for the healthcare system to make proper clinical decisions based on knowledge and data available.[6]
Today, optimism is greater than ever before; the last decade was marked by extraordinary achievements in the field of ML and a broader sense, AI. AI along with advancements in face recognition systems had an impact on every part of society, a healthcare system including the field of orthodontics. The main focus of this narrative review is to provide a detailed as well as a comprehensive summary of AI and ML in the field of orthodontic practice.
UNDERSTANDING VARIOUS TYPES OF AI CLASSIFICATION
AI research to make machines emulate human-like functioning or learning. The degree to which an AI system can replicate human capabilities is the basis for determining various types of AI. Thus, depending on how a machine compares to humans in terms of versatility and performance.
AI can be classified based on functionality and capabilities.
Based on functionality, AI can be classified into four types.[7]
Reactive machines
These are considered to be the oldest forms of AI systems with limited capability that emulates the human mind to respond to various stimuli. These systems do not have memory-based functionality to carry the ability to “learn.” Hence, they are only capable to respond to a combination of inputs or a limited set.
Limited memory
These are machines which are having the capabilities of purely reactive machines, are also capable of learning to make decisions. Nearly, all existing applications belong to this category of AI to store in their memory to form a reference model for solving future problems.
Theory of mind
A theory of mind-level AI will be able to better understand the entities it is interacting with by discerning their needs, emotions, beliefs, and thought processes. This is because to truly understand human needs, AI machines will have to perceive humans as individuals whose minds can be shaped by multiple factors.
Self-aware
Self-aware AI, which, self explanatorily, is an AI that has evolved to be a human brain to develop the function of self-awareness. This type of AI will not only be able to understand and evoke emotions in those it interacts with but also have emotions, needs, beliefs, and potentially desires of its own.
AI can be classified based on capabilities into three basic categories.[8]
Artificial narrow intelligence: This type of AI represents the most complicated and capable to perform a specific task autonomously using human-like capabilities. These machines are programmed, thus have a limited or narrow range of competencies, which is also known as “Weak” AI[9]
Artificial general intelligence: This type of AI system can learn, perceive, understand, and function completely like a human. These systems have multifunctional capabilities that help to build multiple competencies and form connections and generalizations across domains reducing the need for time to perform, which is, therefore, known as “strong” AI[9]
Artificial superintelligence: This system has the capabilities of replicating the multifaceted intelligence of human beings, with greater memory, faster processing, and analysis, as well as decision-making capacity.[8,9]
Based on problem-solving ability, AI is classified into two separate groups. They are symbolic AI and ML. Symbolic AI focused on structuring the algorithm in such a way that it enables humans to understand the data as well as provide details in a readable manner.[10] This technique uses certain rules such as if-then statements, in a situation where certain criterion is met. However, in the medical field, where various conditions or pathologies tend to be more complex and vary between patients to patients wherein systems or models are difficult to works based on various rules and details.[11]
AI methodologies are to identify various pathological conditions of the oral cavity that may go unnoticed.[12]
The AI methodologies include: [Figure 1].

- Artificial intelligence methodologies.
ANNs
It is an efficient data-driven modeling tool that helps in nonlinear dynamic modeling and identification that is having the capability to mimic human brains.[13] It can handle various multivariate parameters which also help in the diagnosis of various systemic or pathologies and also image as well as data analysis.[14]
An important aspect of ANNs includes (1) dilated convolutional neural networks (DCNNs) that focus on sematic scene segmentation. (2) CNNs focus on image classification.
In dentistry, ANN analysis can be used to create a precise prediction system or model which helps to correlate the relation between tooth pain and brushing, the experience of tooth brushing instruction, dental flossing, the replacement cycle of a toothbrush, receiving supragingival scaling, or not, and other factors such as nutrition and exercise of the individual.[15] As a result, a predictive model between pain with teeth with the fitness of 80% could be generated. This system also identifies various factors in preventing pain and also the importance of extraction during orthodontic therapy.[16]
Genetic algorithms
It is the optimization methods for complex problems that give importance to the theory of natural selection. It works on the principle of problem-solving rather than the concept of the analytical relation of conventional methods.[17] This method is important in the regulation of the reconstructed surface and also to maintain the overall smoothness.[18]
Fuzzy logic
Its method of reasoning that resembles human reasoning. It works on the levels of possibilities of input to achieve a definite output. The parts include Rule Base, Fuzzification, and Inference Engine.[17]
Swarm intelligence
Here, each agent follows some simple rules and interacts with the others to share information to lead the behavior to convergence. The most popular algorithms in the research field of swarm intelligence are the ant colony optimization algorithms and the particle swarm optimization algorithms.[19]
Other methods
It includes simulated annealing and evolutionary computing. In the case of simulated annealing which is an iterative search method that starts with an initial solution armed with adequate perturbation and evaluation functions, the algorithm performs a stochastic partial search of the state space, whereas evolutionary computing is a subfield of AI which can produce highly optimized solutions in a wide range of problem settings based on biological evolution.[20]
ML ALGORITHMS
ML algorithms are the system that adjusts themselves to perform betters so that those programs change, how data are processed over time. In the ML mechanism, neurons link the input to give accurate and precise output, where each neuron is linked at the synaptic region like a human neural system. In each synapse, weighted values are adjusted through iterative learning [Figure 2].
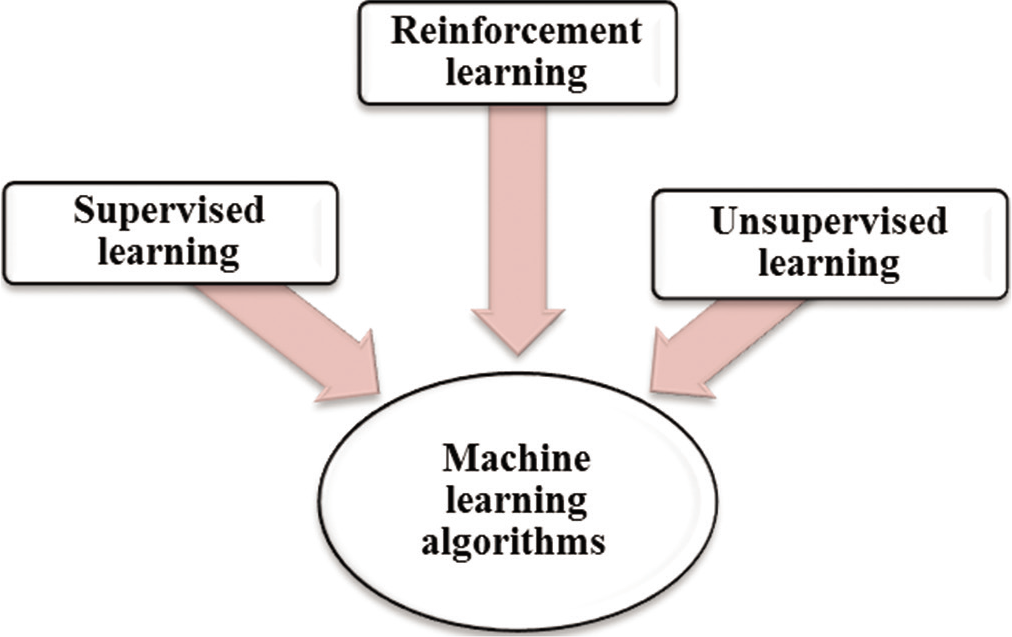
- Machine learning algorithms.
ML algorithms can be divided into three categories, based on the desired outcome and the nature of learning [Figure 3].
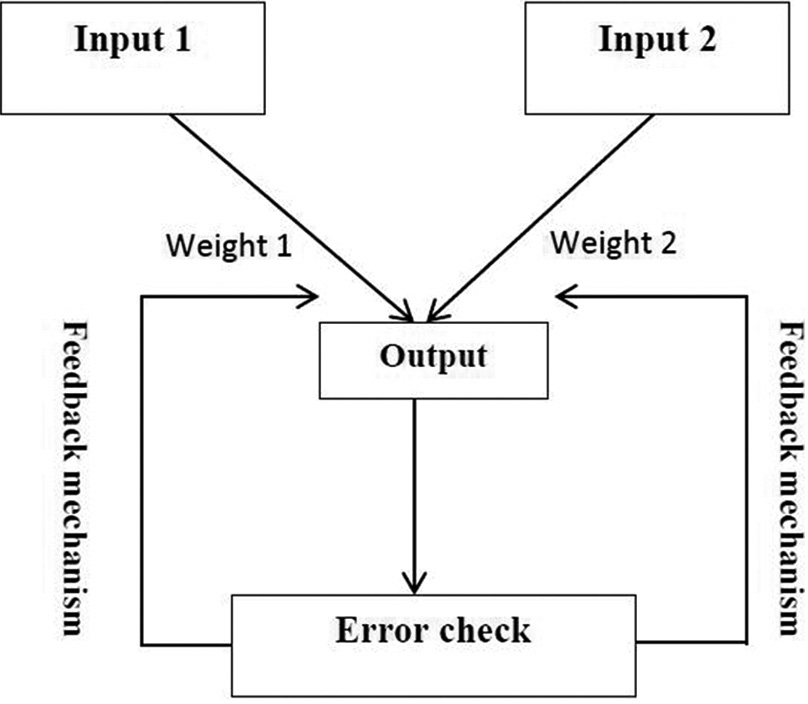
- Machine learning and weighting adjustment.
Supervised learning
This helps to classify the data based on an expected outcome where the prediction model is formed using various variables such as dependent and independent variables.[21] Examples of the algorithm include:
Linear regression for regression problems
Random forest for classification and regression problems
Support vector machine for classification problem.
Unsupervised learning
This method focused to identify the data to find more reasoning information. This requires only a set of input data to group and identify patterns in the data.[21] This allows marketers to develop programs by clustering based on features and similar interests.[22]
Some popular examples of unsupervised learning algorithms are as follows:
k-means for the clustering problem
Apriori algorithm for association rule learning problems.
Reinforcement learning
This type of learning system is supported with a feedback signal. However, the feedback works based on interaction with the dynamic environment.[21] There are two kinds of reinforcement learning, they are positive and negative. Positive is defined as an event that occurs because of specific behavior whereas negative is defined as strengthening of behavior that helps to define the minimum stand of performance.
AI IN HEALTH CARE
The scope of AI in various sectors of the medical field provides a new transfiguring to obtain expert-level information, with the fact that modern medical systems facing difficulties to diagnose and treat various conditions.[23] With the use of modern algorithms, accuracy can be improved in diagnosis, treatment, and care.[24]
AI-based systems in the medical field allow the systematic and structured collection of data of patients that facilitate effective care, this system also helps in reducing the costs of the procedure by minimizing basic or routine tasks. It also facilitates research and development by introducing in silico experimentation options.[25]
AI AND ORTHODONTICS
In the field of orthodontic specialty, although the application of AI is in the initial phases, still it is progressing remarkably. Early attempts to use AI were based on the knowledge-based expert system.[26-29] The future of orthodontic practice may be completely dependent on a digital system, with orthodontists developing neural programs with orthodontic decision-makers to aid in the development of AI for patients to take fewer visits, to reduce treatment duration, and improve the quality of treatment. It would be advantageous for orthodontic specialists to become acquainted with advanced user control interfaces relevant to moving forward into a purely digitalized future.[30]
Dhaimade et al., in 2017, proposed that the culmination of AI along with digitization has seen a new era in the field of dentistry and its future aspects appear extremely promising. [30] Whereas a study by Takada et al., in 2016, stated that AI expert systems with neural network machine learning may assist decision making for orthodontics with improved performance, that is achieved by components such as (1) proper selection of the input data, (2) appropriate organization of the modeling, and (3) preferable generalization.[31]
Collaborating with computer scientists and developing orthodontic appliances tested and validated using various programs can help develop AI in orthodontics. Nowadays, practitioners have more advanced ML systems as a tool in various complicated orthodontic cases with precision and accuracy.[32] These advanced systems are also effective in evaluating post-treatment outcomes and growth changes.
AI FOR ORTHODONTIC DIAGNOSIS AND TREATMENT PLANNING
One of the difficulties faced during orthodontic treatment planning is the decision to extract or not especially in borderline cases.[33] In this concern, efficiently trained neural networks with decision support systems through pre-training can be a precious present to diagnosticians, particularly in cases with multifactorial origins.[34]
Today, these systems or models completely ignore the existence of various pathologic conditions of the oral cavity and treatments that could affect the prescription of orthodontic corrections. This is true in cases with either aligners or fixed appliances therapy.[35] ANNs are one of the futuristic methods that have been successful at predicting the decision to extract in borderline cases and also the need for anchorage.[36,37]
Recently, to determine the forces needed to align teeth and the geometry of various springs, decision support systems were developed as a tool to give precise information but neither system has been used clinically.[38] Another orthodontic challenge during treatment planning is predicting the size of unerupted teeth. To address this, a hybrid system using ANN and genetic algorithms was used to predict canine and premolar sizes.[39]
These AI-based system assists orthodontists to determine orthodontic tooth movement but there are several limitations of ML in traditional clear aligner therapy.[40] Another limitation of the system is that they do not involve the importance of facial analysis, their proportions, and the esthetics of individuals.[41,42]
Neural networks were also used to estimate the dental ages of the patient from panoramic radiographs. According to it, the root means square error (RMSE) was about 0.9 for girls whereas, in the case of boys, it is 1.1, while traditional regression had an RMSE of 1.3 girls and 1.4 for boys.[43] In the orthodontic treatment system, to predict the position impacted maxillary canine based on linear and angular measures, various radiographic X-ray images such as lateral cephalometric and orthopantamograph have been used widely. The random forest algorithm is the one that shows the greatest prediction accuracy about 88.3%.[44]
Selection of the appropriate treatment method and appliance system is one of the difficulties of the main challenges faced by less experienced orthodontists nowadays. To manage this, newer algorithms are being developed that will support to choose appliances like headgears.[45]
Kok et al. studied different AI algorisms for the determination or prediction of growth by cervical vertebrae maturity stages. Accuracy can be tested by K-nearest neighbors, Naive Bayes, decision trees, etc. ANN showed the most stable result and was suggested as the preferred method for determining the cervical vertebrae stage.[46]
AI AND ORTHODONTIC TREATMENT OUTCOMES
With the latest advancement and precision of AI, it is very useful in predicting the soft-tissue outcomes of orthodontic treatment which is considered to be paramount in the final stability of treatment. Especially in complicated cases of skeletal Class II and Class III, individual precise predictions of treatment outcomes have been reported.[30] Using ANN, facial attractiveness was quantified and scored at different levels of facial attractiveness and apparent age of orthognathic patients.[47]
Recently, ANN was used to predict the change in lip curvature after orthodontic treatment with or without extractions.[48] Its prediction of change and the actual change that occurred differed by 29.6% and 7% for the upper and lower lips, respectively. Both the predictions were much better than those based on linear regression. The system was then applied to a treated sample, revealing that all unsuccessful cases belonged to either the hypermandibular or hyperdivergent cluster. Another system correctly predicted the outcome of Class III treatment 97.2% of the time, which was slightly higher than the 92.1% reported for discriminant analysis.[49]
VARIOUS OTHER APPLICATIONS OF AI
According to Shoukri et al., the neural network can be used to classify or stage changes in condylar morphology in various degrees of temporomandibular joint osteoarthritis.[46] Predictive analytical staging showed 73.5% and 91.2% accuracy of TMJOA compared to the repeated practice consensus which gives the importance of AI in the classification of TMD.[50]
Another important application is in the case of cleft studies. According to the studies of Zhang et al., AI validates the diagnostic effectiveness of 13 single-nucleotide polymorphisms (13-SNPs) that have been detected using various genome-wide association studies which have been conducted earlier. Different ML algorisms had been used to evaluate prediction performance and build predictive models with those SNPs. The result of these studies identified logistic regression as best for evaluating or analyzing risk assessment.[51]
AI is very effective in image segmentation and landmark identification. Image segmentation helps to isolate the pixels of the target lesion or conditions from X-rays, CT, or MRI which, in turn, helps in automated or semi-automated computer-aided diagnosis systems, and it is also important in volumetric medical image analysis which is mostly done with the Dice ratio.[52] According to the study of Wang et al., CBCT was used to develop an effective method for automated segmentation of maxilla and mandible by learning-based based on random forest.[53]
Last but not least, thanks to the power of technology, diagnostic and therapeutic philosophy are going through a paradigm shift from the traditional “signs and symptoms” approach to the “precision medicine” approach.[54] Despite the potential for various concerns about patient privacy and misinterpretation of data, AI and ML will continue to connect the specialty of orthodontics from a comprehensive perspective and form the walk toward a better future.
CONCLUSION
AI, as well as ML, is not just a myth or imagination, it is the present and future of the healthcare system including the field of orthodontics. Over the past few years, applications of AI are growing day by day at a rapid pace. While the system of AI is not there to replace the role of humans, in treating various healthcare conditions including craniofacial defects, it is an important tool that will support and help them in various stages of practice including diagnosis of disease, treatment planning, and predicting the outcome of treatment. Nevertheless, it is important to have in-depth knowledge about various concepts and the fact of AI that will be a great benefit in the present and coming future.
Declaration of patient consent
Patient consent is not required as there are no patients in this study.
Financial support and sponsorship
Nil.
Conflicts of interest
There are no conflicts of interest.
References
- Maxillary arch width and buccal corridor changes with Damon and conventional brackets: A retrospective analysis. Angle Orthod. 2016;86:655-60.
- [CrossRef] [PubMed] [Google Scholar]
- Comparison of changes in the dental transverse and sagittal planes between patients treated with self-ligating and with conventional brackets In: Dental Press J Orthod. Vol 25. 2020. p. :47-55.
- [CrossRef] [PubMed] [Google Scholar]
- Artificial Intelligence: A New Synthesis In: Massachusetts. United States: Morgan Kaufmann Publishers Inc; 1998. p. :513.
- [Google Scholar]
- An application of belief merging for the diagnosis of oral cancer. Appl Soft Comput J. 2017;61:1105-12.
- [CrossRef] [Google Scholar]
- Artificial intelligence in dentomaxillofacial radiology. Acta Sci Dent Sci. 2019;3:116-21.
- [Google Scholar]
- Clinical decision support: Strategies for success. Procedia Comput Sci. 2014;37:422-7.
- [CrossRef] [Google Scholar]
- Artificial intelligence (AI) or intelligence augmentation (IA): What is the future? AI. 2020;1:143-55.
- [CrossRef] [Google Scholar]
- Will artificial intelligence solve the human resource crisis in healthcare? BMC Health Serv Res. 2018;18:545.
- [CrossRef] [PubMed] [Google Scholar]
- Distinguishing between Narrow AI. 2018. General AI and Super AI. Available from: https://www.medium.com/@tjajal/distinguishing-between-narrow-ai-general-ai-and-super-ai-a4bc44172e22 [Last accessed on 2019 Dec 07]
- [Google Scholar]
- Artificial General Intelligence Berlin, Heidelberg: Springer-Verlag Berlin Heidelberg; 2007.
- [CrossRef] [PubMed] [Google Scholar]
- Artificial intelligence in medicine. Where do we stand? N Engl J Med. 1987;316:685-8.
- [CrossRef] [PubMed] [Google Scholar]
- Artificial intelligence: A dentist's perspective. J Med Radiol Pathol Surg. 2018;5:2-4.
- [CrossRef] [Google Scholar]
- A logical calculus of the ideas immanent in nervous activity. Bull Math Biol. 1990;52:99-115.
- [CrossRef] [Google Scholar]
- Investigating CDSS success factors with usability testing. Int J Adv Comput Sci Appl. 2017;8:548-54.
- [CrossRef] [Google Scholar]
- Predictive modeling of dental pain using neural network. Stud Health Technol Inform. 2009;146:745-6.
- [Google Scholar]
- Data mining of clinical oral health documents for analysis of the longevity of different restorative materials in Finland. Int J Med Inform. 2009;78:68-74.
- [CrossRef] [PubMed] [Google Scholar]
- Present and future of artificial intelligence in dentistry. J Oral Biol Craniofac Res. 2020;10:391-6.
- [CrossRef] [PubMed] [Google Scholar]
- Genetic algorithms based approach for dental caries detection using back propagation neural network. Int J Recent Technol Eng. 2019;8:1-4.
- [Google Scholar]
- Solving the traveling salesman problem based on the genetic simulated annealing ant colony system with particle swarm optimization techniques. Expert Syst Appl. 2011;38:14439-50.
- [CrossRef] [Google Scholar]
- Evolutionary algorithms, simulated annealing and tabu search: A comparative study. Eng Appl Artif Intell. 2001;14:167-81.
- [CrossRef] [Google Scholar]
- Applications of artificial intelligence and machine learning in orthodontics. APOS Trends Orthod. 2020;10:17-24.
- [CrossRef] [Google Scholar]
- Right Care Shared Decision Making Programme, Capita. In: Measuring Shared Decision Making: A Review of Research Evidence NHS. 2012.
- [Google Scholar]
- The potential for artificial intelligence in healthcare. Future Healthc J. 2019;6:94-8.
- [CrossRef] [PubMed] [Google Scholar]
- On the prospects for a (deep) learning health care system. JAMA. 2018;320:1099-100.
- [CrossRef] [PubMed] [Google Scholar]
- A computer-controlled expert system for orthodontic advice. Br Dent J. 1987;163:161-6.
- [CrossRef] [PubMed] [Google Scholar]
- The initial use of a computer-controlled expert system in the treatment planning of Class II Division 1 malocclusion. Br Dent J. 1991;18:1-7.
- [CrossRef] [PubMed] [Google Scholar]
- Artificial intelligence and machine learning: The new paradigm in orthodontic practice. Int J Orthod Rehabil. 2020;11:175-9.
- [CrossRef] [Google Scholar]
- Artificial intelligence: Transforming dentistry today. Indian J Basic Appl Med Res. 2017;6:161-7.
- [Google Scholar]
- Artificial intelligence expert systems with neural network machine learning may assist decision-making for extractions in orthodontic treatment planning. J Evid Based Dent Pract. 2016;16:190-2.
- [CrossRef] [PubMed] [Google Scholar]
- Application of a case-based expert system to orthodontic diagnosis and treatment planning: A review of the literature. Aust Orthod J. 1996;14:150-3.
- [Google Scholar]
- Consistency of orthodontic extraction decisions. Eur J Orthod. 1996;18:77-80.
- [CrossRef] [PubMed] [Google Scholar]
- Artificial neural network modeling for deciding if extractions are necessary prior to orthodontic treatment. Angle Orthod. 2010;80:262-6.
- [CrossRef] [PubMed] [Google Scholar]
- Interest in orthodontic tooth alignment in adult patients affected by periodontitis: A questionnaire-based cross-sectional pilot study. J Periodontol. 2019;90:957-65.
- [CrossRef] [PubMed] [Google Scholar]
- Orthodontic treatment planning based on artificial neural networks. Sci Rep. 2019;9:2037.
- [CrossRef] [PubMed] [Google Scholar]
- Automatic cephalometric analysis: A systematic review. Angle Orthod. 2008;78:145-51.
- [CrossRef] [PubMed] [Google Scholar]
- Decision support system for determination of forces applied in orthodontic based on fuzzy logic. The International Conference on Advanced Machine Learning Technologies and Applications. AMLTA In: Advances in Intelligent Systems and Computing. Vol 723. Cham, Switzerland: Springer; 2018.
- [CrossRef] [Google Scholar]
- Design and implementation of a hybrid genetic algorithm and artificial neural network system for predict ing the sizes of unerupted canines and premolars. Eur J Orthod. 2012;34:480-6.
- [CrossRef] [PubMed] [Google Scholar]
- Artificial intelligence in orthodontics. APOS Trends Orthod. 2019;9:201-5.
- [CrossRef] [Google Scholar]
- Esthetic perception of facial profile contour in patients with different facial profiles. J World Fed Orthod. 2019;8:112-7.
- [CrossRef] [Google Scholar]
- The maxillary incisor display at rest: Analysis of the underlying components In: Dental Press J Orthod. Vol 23. 2018. p. :48-55.
- [CrossRef] [PubMed] [Google Scholar]
- Computer-aided diagnosis of periapical cyst and keratocystic odontogenic tumor on cone beam computed tomography. Comput Methods Programs Biomed. 2017;146:91-100.
- [CrossRef] [PubMed] [Google Scholar]
- Dental age estimation and different predictive ability of various tooth types in the Czech population: Data mining methods. Anthropol Anz. 2013;70:331-45.
- [CrossRef] [PubMed] [Google Scholar]
- Determinants of maxillary canine impaction: Retrospective clinical and radiographic study. J Clin Exp Dent. 2017;9:1304-9.
- [CrossRef] [PubMed] [Google Scholar]
- Usage and comparison of artificial intelligence algorithms for determination of growth and development by cervical vertebrae stages in orthodontics. Prog Orthod. 2019;20:41.
- [CrossRef] [PubMed] [Google Scholar]
- Applying artificial intelligence to assess the impact of orthognathic treatment on facial attractiveness and estimated age. Int J Oral Maxillofac Surg. 2019;48:77-83.
- [CrossRef] [PubMed] [Google Scholar]
- Artificial neural network (ANN) modeling and analysis for the prediction of change in the lip curvature following extraction and non-extraction orthodontic treatment. J Dent Spec. 2015;3:217-9.
- [CrossRef] [Google Scholar]
- Artificial Intelligence: A Modern Approach (3rd ed). Upper Saddle River, New Jersey: Pearson Education; 2011.
- [Google Scholar]
- Minimally invasive approach for diagnosing TMJ osteoarthritis. J Dent Res. 2019;98:1103-11.
- [CrossRef] [PubMed] [Google Scholar]
- Machine learning models for genetic risk assessment of infants with non-syndromic orofacial cleft. Genomics Proteomics Bioinformatics. 2018;16:354-64.
- [CrossRef] [PubMed] [Google Scholar]
- Deep learning techniques for medical image segmentation: Achievements and challenges. J Digit Imaging. 2019;32:582-96.
- [CrossRef] [PubMed] [Google Scholar]
- Automated segmentation of dental CBCT image with prior-guided sequential random forests. Med Phys. 2016;43:336-46.
- [CrossRef] [PubMed] [Google Scholar]
- Moving towards precision orthodontics: An evolving paradigm shift in the planning and delivery of customized orthodontic therapy. Orthod Craniofac Res. 2017;20(Suppl 1):106-13.
- [CrossRef] [PubMed] [Google Scholar]