Translate this page into:
Applying artificial intelligence to predict the outcome of orthodontic treatment
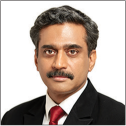
*Corresponding author: Krishnaswamy Nathamuni Rengarajan, Department of Orthodontics, Ragas Dental College and Hospital, Chennai, Tamil Nadu, India. nrkorthodontics@gmail.com
-
Received: ,
Accepted: ,
How to cite this article: Ramasubbu N, Valai Kasim S, Thavarajah R, Nathamuni Rengarajan K. Applying artificial intelligence to predict the outcome of orthodontic treatment. APOS Trends Orthod. 2024;14:264-72. doi: 10.25259/APOS_270_2023
Abstract
Objectives:
The study aimed to train an algorithm to predict facial and dental outcomes following orthodontic treatment using artificial intelligence (AI). In addition, the accuracy of the algorithm was evaluated by four distinct groups of evaluators.
Material and Methods:
The algorithm was trained using pre-treatment and post-treatment frontal smiling and intraoral photographs of 50 bimaxillary patients who underwent all first bicuspid extraction and orthodontic treatment with fixed appliances. A questionnaire was created through Google form and it included 10 actual post-treatment and AI-predicted post-treatment images. The accuracy and acceptability of the AI-predicted outcomes were analyzed by four groups of 140 evaluators (35 orthodontists, 35 oral maxillofacial surgeons, 35 other specialty dentists, and 35 laypersons).
Results:
The Style-based Generative Adversarial Network-2 algorithm used in this study proved effective in predicting post-treatment outcomes using pre-treatment frontal facial photographs of bimaxillary patients who underwent extraction of all first bicuspids as part of their treatment regimen. The responses from the four different groups of evaluators varied. Laypersons exhibited greater acceptance of the AI-predicted images, whereas oral maxillofacial surgeons showed the least agreement. The base of the nose and the chin demonstrated the most accurate predictions, while gingival visibility and the upper lip-to-teeth relationship exhibited the least prediction accuracy.
Conclusion:
The outcomes underscore the potential of the method, with a majority of evaluators finding predictions made by the AI algorithm to be generally reliable. Nonetheless, further research is warranted to address constraints such as image tonicity and the proportional accuracy of the predicted images.
Keywords
Artificial intelligence
Style-based Generative Adversarial Network-2 algorithm
Orthodontic treatment
Facial and dental outcomes
Prediction
INTRODUCTION
Bimaxillary protrusion is a prevalent malocclusion characterized by proclined incisors and increased protrusion of the lips.[1,2] The primary objective of orthodontic treatment is to retract the upper and lower incisors, resulting in improved soft-tissue procumbency and convexity.[3,4] This is frequently achieved through the extraction of the first bicuspid. However, the notion of extraction is often met with skepticism among patients due to concerns that it may adversely affect facial esthetics. Patients also commonly express anxiety regarding the facial outcomes following the first bicuspid extraction.
Conventionally, predictions of facial and dental treatment outcomes were based on lateral cephalograms.[5,6] In recent times, computerized cephalometric systems such as the Dolphin imaging system, Materialise Mimics, and similar programs have emerged for this purpose. However, these methods have limitations as they can only forecast changes in the profile view and often struggle to accurately predict changes in the frontal view. Moreover, they rely on manual annotations and their accuracy in the lower third of the face has been subject to scrutiny.[7,8]
Given these limitations, an alternative method has been developed using artificial intelligence (AI). In computer science, AI refers to the ability to mimic cognitive functions associated with humans, such as learning and problem-solving. One subfield of AI is neural networks, which are mathematical computing models that simulate the functioning of the human brain.[9] These networks can be trained with clinical data and utilized for various tasks in the field of orthodontics. Convolutional neural networks (CNNs), in particular, have shown exceptional performance in image recognition and classification.[10] These models are biologically inspired and often mimic vision processing in living organisms. Recently, AI has garnered significant attention for predicting facial outcomes following orthodontic treatment and orthognathic surgery. With the advancement of AI, new opportunities for image processing and task automation has emerged.
The present investigation aimed to utilize AI as an aid in developing an algorithm Style-based Generative Adversarial Network-2 (StyleGAN-2) for predicting frontal facial and dental outcomes following orthodontic treatment, using pre-treatment photographs. The primary objective was to train the algorithm to predict frontal facial and dental outcomes of bimaxillary patients post-treatment. The secondary objective was to analyze the AI-predicted post-treatment outcomes, which were evaluated by four different groups of evaluators.
MATERIAL AND METHODS
This retrospective longitudinal cohort study was conducted at a local university, utilizing records obtained from the archives of the Department of Orthodontics. The study included fifty bimaxillary protrusion patients (18 males and 32 females) who underwent all first bicuspid extraction and received orthodontic treatment with fixed orthodontic appliances as part of their treatment plan. Sample size calculation for the training and testing datasets was performed using G*Power version 3.0, with an alpha error of 0.20 and a power of 0.95. The total sample size was calculated as 48, which was rounded up to 50 for practicality. Institutional Review Board approval (no. 20210405) was obtained for this study, ensuring patient anonymity and obtaining written consent from all participants.
The patient records consisted of pre-treatment and post-treatment frontal smiling and intraoral images of sufficient diagnostic quality. Subjects aged between 18 and 30 years (mean age: 19.4 ± 3) were included, exhibiting a Class I skeletal and dental pattern (Angle determined by points A, N and B [ANB] of 2–4°) and proclined incisors with mild to moderate crowding. Exclusion criteria encompassed patients with severe crowding exceeding 6 mm, open-bite cases, skeletal class II and III patterns, a history of previous orthodontic treatment or orthognathic surgery, facial trauma, cosmetic surgery, facial asymmetries, and clefts, as well as inadequate diagnostic records.
All images were captured using a Digital Single Lens Reflex camera (Canon - USA) equipped with a 100 mm macro lens, a dedicated flash reflector, and a monochrome background. The photographs were standardized to ensure consistent head size across all images. No digital image enhancement, apart from adjustments to contrast and brightness, was applied. The photographs were archived and processed digitally in JPEG format at 300 dpi.
The 50 pre-treatment and post-treatment datasets were split into 40 training sets and 10 testing sets. The StyleGAN-2 algorithm, developed by Nvidia researchers in California, USA, was employed in this study. Generative Adversarial Network is a machine learning framework that generates large, high-quality images. The network training involves feeding samples from the training dataset (pre-treatment and post-treatment frontal smiling images) until it achieves acceptable accuracy. This process enables the algorithm to identify the face, extract facial features, and learn to compare changes in facial patterns between pre-treatment and post-treatment images. Digital Library (DLIB) was utilized to detect key facial features and localize the face in the image. The pre-trained facial landmark detector within DLIB was employed to estimate the location of 68 x and y-coordinates that map the structures on the face [Figure 1].
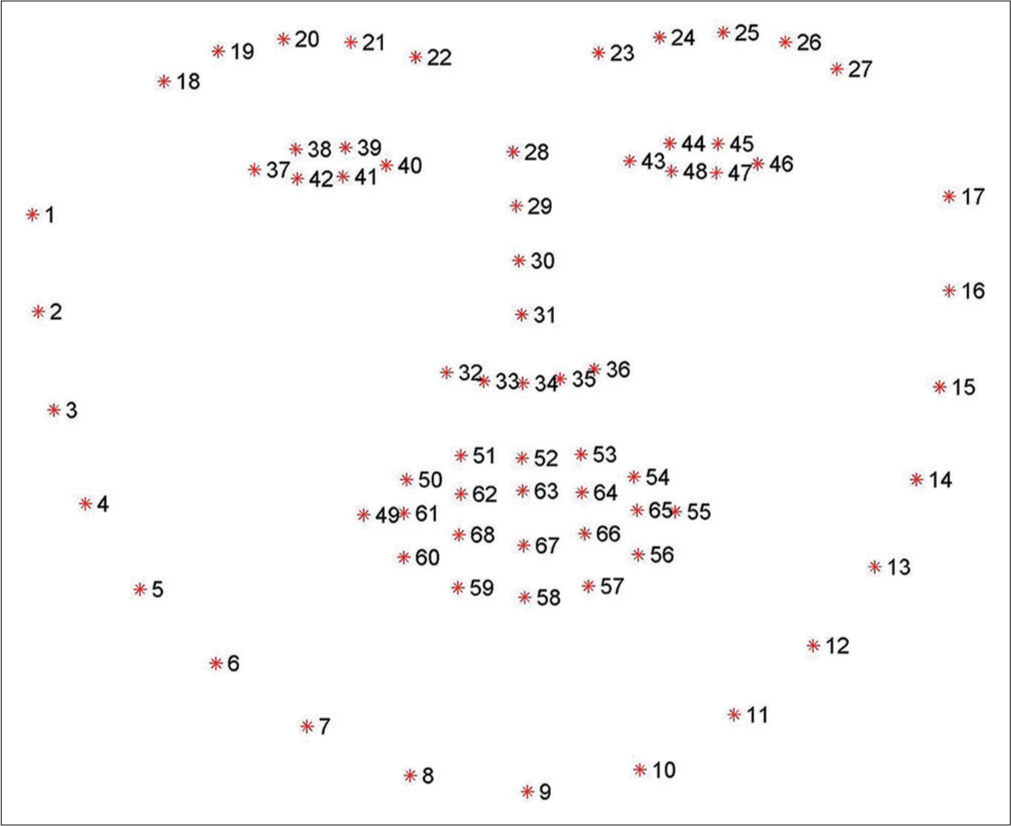
- Facial landmark detection.
Following facial point feature extraction, the Tensorflow framework was activated, introducing nonlinearity in neural networks to create realistic images. Tensorflow verifies all previous processes and corrects any missing features or errors at this stage. Facial alignment, followed by deep learning prediction, yields the desired AI-predicted post-treatment image [Figure 2]. The presented algorithm was effective in generating predicted post-treatment facial and dental outcomes following orthodontic treatment, using pre-treatment photographs. The AI-predicted outcomes were realistic and comparable to the actual post-treatment outcomes.
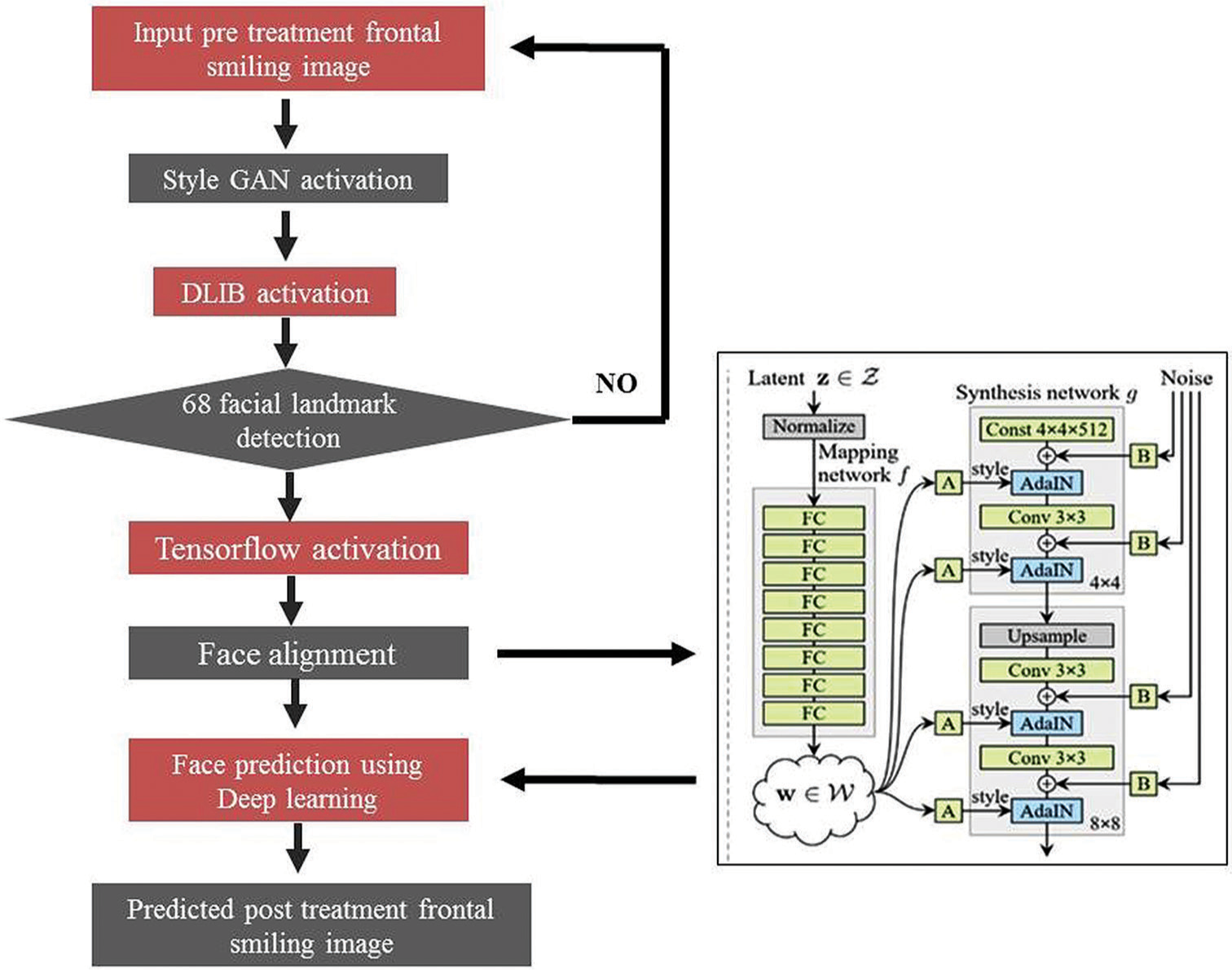
- Summary of the workflow. StyleGAN-2: Style-based Generative Adversarial Network-2, DLIB: Digital Library. FC: Fully connected Layers: In a Generative Adversarial Network (GAN), fully connected layers are often used in the generator and discriminator networks. These layers are used to transform the input data (e.g., noise vector for the generator, real/fake image for the discriminator) into a format that can be used for further processing and decision making. z: is the initial noise vector sampled from a simple distribution, Z: represents the entire input space of the generator, which includes all possible noise vectors z, w: is an intermediate latent space obtained after the FC layer, W: represents the entire intermediate latent space, which includes all possible intermediate vectors w that can be obtained after the FC layer, A: Style Input- style input typically refers to a style image or a learned style representation. B: Noise Input- This is a random noise vector sampled from a simple distribution, such as a Gaussian or uniform distribution AdaIN (Adaptive Instance Normalization): is a technique commonly used in the architecture of Generative Adversarial Networks (GANs) to improve the style transfer capabilities of the generator network. It is introduced in the context of image style transfer in this context it is used for image generation and manipulation, Const 4x4x512: refers to a constant tensor or array with dimensions 4x4 and a depth or number of channels equal to 512. This is commonly encountered in the generator network of a GAN, where it represents a fixed, learned representation that is used as input or as a starting point for generating images. Latent z ∈ Z represents a randomly sampled vector from a simple distribution (Gaussian distribution).This vector serves as the input to the generator network and is referred to as the latent code.” It captures the random variations that the generator uses to produce diverse outputs. Latent space w ∈ W represents an intermediate latent space that has been linearly transformed from z and is used to modulate the activations of the generator network. This transformation is often done to disentangle the latent factors of variation in the input, making the learned representation more interpretable and enabling finer control over the generated outputs.
A Google form was utilized to craft a questionnaire [Table 1] featuring 10 (4 males and 6 females) randomly selected actual post-treatment and AI-predicted post-treatment images. Alongside the actual and predicted post-treatment frontal images, the patient’s pre-treatment frontal image was provided as a point of reference for comparison. The images were cropped to eye level to minimize distractions for the evaluators. Subsequently, the accuracy and acceptability of the predicted outcomes were analyzed.
S. No. | Questions included |
---|---|
1. | To recognize the actual post treatment image and the AI predicted post treatment image. |
2. | To rate the similarities in terms of facial and dental appearance between the actual and the AI predicted post treatment images. |
3. | To find which region of the face was almost identical between the actual and predicted images. |
AI: Artificial intelligence
The sample size for the evaluators who participated in the study was determined using G*Power version 3.0, with an alpha error of 0.20 and a power of 0.95. Based on this calculation, it was determined that 140 evaluators were needed. They were divided into four groups, each comprising 35 evaluators (orthodontists, oral maxillofacial surgeons, other specialty dentists, and laypeople). Primary data concerning the evaluators’ age, gender, profession, and experience were also recorded. All orthodontists, oral maxillofacial surgeons, and other specialty dentists participating in our study were accredited by their respective professional boards. Additionally, the professions of laypersons that could potentially influence their perception of facial symmetry and esthetics, such as artists, photographers, cosmetologists, and beauticians, were excluded to minimize bias.
Statistical analysis
Statistical Package for the Social Sciences software (Version 26.0; IBM Corp., Armonk, New York, USA) was employed for data analysis. Reliability and validity tests were conducted for all questionnaire items. The data were assessed for normality using the Shapiro-Wilk test, indicating a normal distribution (>0.05). Descriptive statistics were utilized to elucidate the variations in the percentage distribution of responses by evaluators for all 10 pairs of images (actual post-treatment and AI-predicted post-treatment) in the questionnaire. To ascertain differences in opinion among the four evaluator groups and to determine whether age, gender, and experience influenced responses, the Chi-square test was employed. Mean differences were calculated using unpaired/independent sample t-tests to compare perceptions of actual and AI-predicted images between laypersons and dentists. The chosen significance level for all statistical tests was P < 0.05.
RESULTS
The StyleGAN-2 algorithm utilized in this study demonstrated its efficacy in generating anticipated post-treatment facial and dental outcomes in the frontal dimension based on pre-treatment photographs of bimaxillary patients who underwent all first bicuspid extraction and orthodontic treatment with fixed appliances.
The reliability and validity tests for the questionnaire yielded a Cronbach’s Alpha value of 0.914 (>0.9 - excellent) and 0.367 (>0.35 - very beneficial), respectively, which were considered favorable for the research. Descriptive data for the four groups of evaluators included in the study are provided in [Table 2].
Variables | Categories | n (%) |
---|---|---|
Gender | Male | 78 (56) |
Female | 62 (44) | |
Age | <30 years | 40 (29) |
31–40 years | 51 (36) | |
41–50 years | 35 (25) | |
>50 years | 14 (10) | |
No. of years of experience after post-graduation | <5 years | 39 (28) |
5–10 years | 46 (33) | |
10–20 years | 35 (25) | |
>20 years | 20 (14) |
Inference obtained from the questionnaire
Question 1 – To recognize the actual post-treatment image and the AI-predicted post-treatment image.
Orthodontists, other specialty dentists, and laypersons successfully identified the AI-predicted and actual post-treatment images in seven out of ten pairs. Conversely, oral maxillofacial surgeons recognized only five out of ten image sets [Figure 3]. The majority of evaluators struggled to distinguish between the AI-predicted and actual images in the fifth pair included in the questionnaire [Figure 4].

- Representation of question 1 (Q1) – To recognize the actual post treatment image and AI predicted post-treatment image; and question 2 (Q2) – To rate the similarities in terms of facial and dental appearance between the actual and AI predicted treatment images. The scale represents the image sets in the form of percentage (0–100%). The red and green color indicates the responses of the evaluators for the Q1 and Q2, respectively.
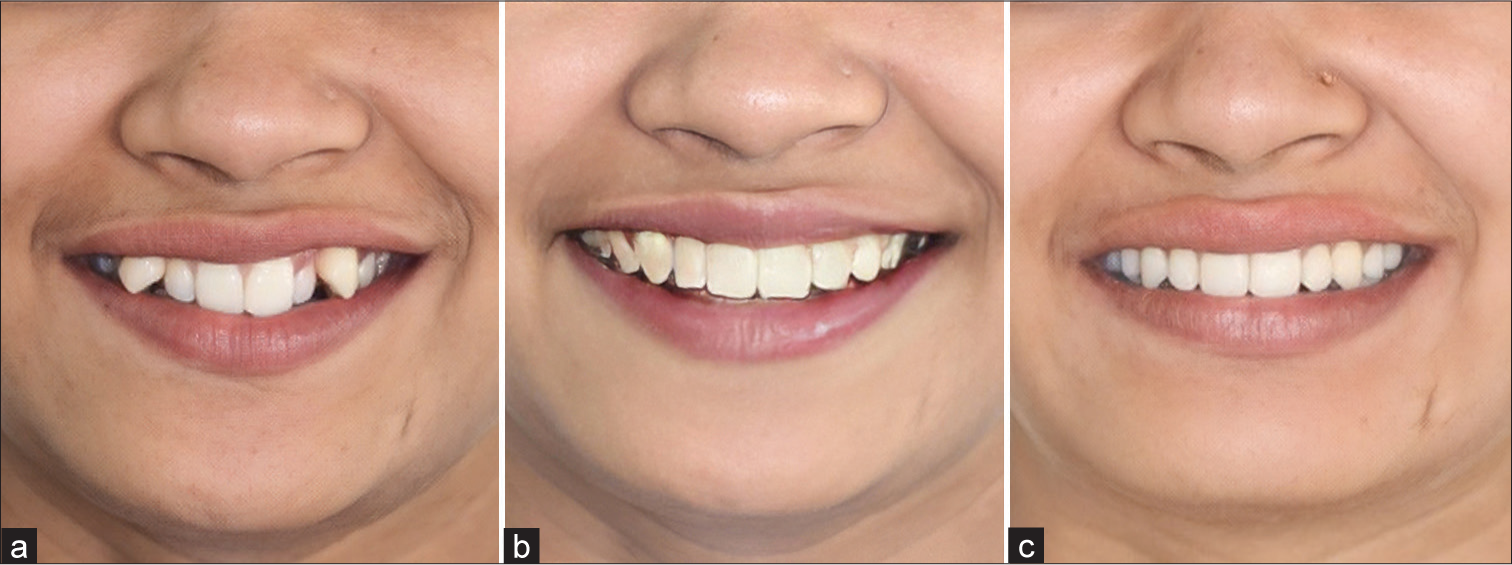
- Artificial intelligence (AI) predicted image with greatest accuracy. (a) Pre-treatment image used for comparison. (b) AI predicted post-treatment image. (c) Actual post-treatment image.
Question 2 – To rate the similarities in terms of facial and dental appearance between the actual and the AI-predicted post-treatment images.
The layperson responses revealed that six out of ten pairs of images exhibited similar facial and dental appearances between the actual and AI-predicted images. Conversely, orthodontists and other specialty dentists found similarities among five pairs of images, while oral maxillofacial surgeons acknowledged similarities in only two pairs. Nearly all evaluators reached a consensus regarding the fifth pair, affirming significant facial and dental similarities. Conversely, unanimous disagreement was noted among all evaluators regarding the first pair [Figure 5]
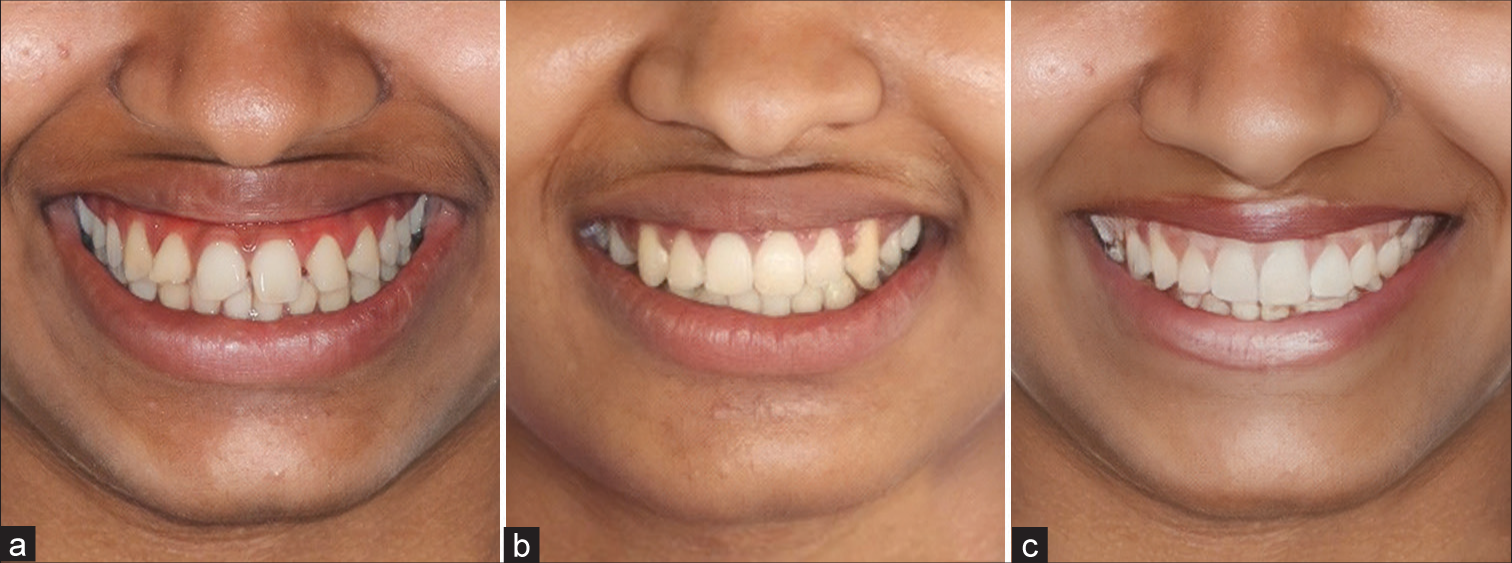
- Artificial intelligence (AI) predicted image with least accuracy. (a) Pre-treatment image used for comparison. (b) Actual post-treatment image. (c) AI predicted post-treatment image.
Question 3 – To find which region of the face was almost identical between the actual and AI-predicted post-treatment images.
All four groups unanimously identified the base of the nose and chin as the most identical regions between the actual and AI-predicted images. Conversely, the least identical regions were determined to be the gingival visibility and the relationship between the upper lip and teeth [Figure 6].
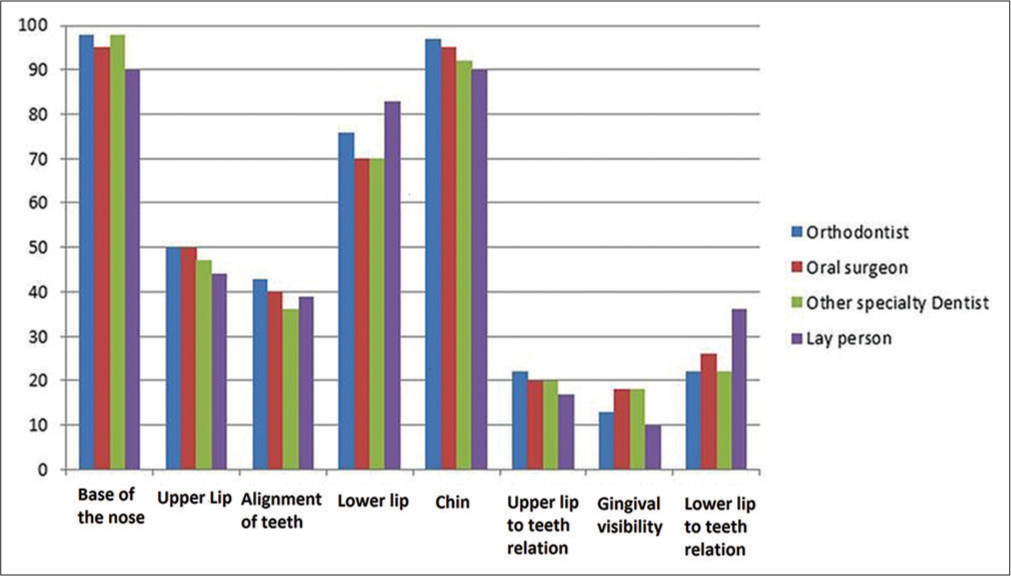
- Representation of question 3 – To find which region of face was identical between the actual and the artificial intelligence (AI) predicted between the actual and AI predicted images.
The inter-rater reliability was assessed by randomly selecting 10 evaluators from each group, and their responses were recorded for the same questionnaire after a 10-day interval. Upon comparing the responses at baseline and after the 10 days, Cohen’s kappa statistic was calculated to be 0.83, indicating near-perfect agreement among independent observers with no significant variation. In addition, the Chi-square test revealed differences in responses among the four groups of evaluators. Furthermore, factors such as evaluators’ age, gender, and experience showed no significant effect on their responses.
The mean difference, determined through unpaired sample t-tests, highlighted that the level of acceptance of AI-predicted images by laypersons was higher than that of dentists (other specialty dentists, orthodontists, and oral maxillofacial surgeons).
DISCUSSION
Predicting the facial and dental treatment outcomes has always presented a challenge, despite being of considerable interest to both orthodontists and patients. Historically, treatment predictions relied on conventional cephalograms or 2D facial images, which often fell short in forecasting outcomes in the frontal dimension. The feasibility of predicting post-treatment outcomes from pre-treatment frontal images appeared promising using AI. Thus, this study was conceived to generate post-treatment outcomes from pre-treatment frontal photographs of bimaxillary patients undergoing treatment with fixed orthodontic appliances following first bicuspid extractions.
The hypothesis of this study aimed to determine the possibility of predicting post-treatment frontal facial and dental appearances using AI and to assess whether the predictions were accurate enough for clinical deployment.
Only a handful of studies have endeavored to assess treatment outcomes using AI. For instance, Patcas et al.[11] employed a convolutional neural network (CNN) to evaluate changes in facial attractiveness in patients undergoing orthognathic surgery. Their CNN underwent minimal processing compared to other image-classifying networks reliant on hand-engineered algorithms.[11] Similarly, Tanikawa and Yamashiro Takashi et al. developed two distinct algorithms utilizing AI-driven deep learning methods to predict facial shape alterations following orthodontic treatment (first premolar extraction) and orthognathic surgery.[12] This suggests the potential for AI as a reliable tool for outcome prediction before commencing orthodontic treatment. However, it’s worth noting that Tanikawa’s study was constrained to predicting 3D facial topography post-orthodontic treatment and orthognathic surgery, subsequently assessing prediction errors. In our investigation, the algorithm was designed to generate realistic post-treatment frontal facial and dental images of bimaxillary patients undergoing first bicuspid extraction alongside fixed appliance therapy.
In the present study, sample size calculation was performed with an alpha error of 0.20 and a power of 0.95, indicating that a sample size of 50 would yield sufficient data for training and testing the AI algorithm. This aligns closely with the findings of Tanikawa and Yamashiro,[12] whose study utilized a sample size of 65. The algorithm employed in our research, StyleGAN-2, has been validated for its efficacy in generating large, high-quality images. Karras et al. demonstrated that the StyleGAN-2 model not only produces photorealistic images of faces, but also provides greater control over the style of the generated image at various levels of detail by manipulating style vectors and noise.[13]
In our study, the algorithm effectively predicted the post-treatment facial and dental outcomes of bimaxillary patients who underwent extraction of all first bicuspids, followed by the application of fixed appliances. However, on comparing the actual and AI-predicted post-treatment images, minor issues were observed regarding image tonicity and proportions. The enhanced image tonicity present in the AI-predicted images was inadvertently incorporated into the algorithm during the coding process, necessitating correction to achieve raw images with natural tonicity. The discrepancy in image proportionality may be attributed to the stretching of the image in horizontal or vertical dimensions during data collection or processing stages of the algorithm.
The validity of the included questionnaire was deemed beneficial for this research, as determined by Cronbach’s test.[14] Kokich et al. utilized photographs and questionnaires to evaluate the perceptions of laypeople, orthodontists, and general dentists regarding variations in anterior tooth size, alignment, and their relation to the surrounding soft tissues.[15] Similarly, in our study, photographs and questionnaires were employed to compare the AI-predicted treatment outcomes with the actual post-treatment outcomes. Dourado et al.[16] conducted a study to assess facial pleasantness using the Likert scale and the visual analog scale (VAS). When evaluators, including orthodontists, oral maxillofacial surgeons, and laypeople, were asked to assess photographs of patients seeking orthodontic treatment, they expressed a preference for the Likert scale over the VAS due to its simplicity.[16] Consequently, the present study also incorporated a 5-point Likert scale as part of the questionnaire.
In a study by Patcas et al., AI and 39 human evaluators (comprising 14 orthodontists, 10 oral maxillofacial surgeons, and 15 laypeople) were employed to assess the facial attractiveness of post-treatment cleft patients using facial photographs.[17] In our study, following a power of 0.95, 140 evaluators were divided into four equal groups (35 orthodontists, 35 oral maxillofacial surgeons, 35 other specialty dentists, and 35 laypeople) tasked with evaluating both the actual and AI-predicted post-treatment images.
When the evaluators were asked to rate the similarities in terms of facial and dental appearance between the actual and the AI-predicted images using a 5-point Likert scale, all the groups except oral maxillofacial surgeons revealed that more than 50% of the actual and AI-predicted images were almost identical. This suggests that oral maxillofacial surgeons demonstrated a higher level of discernment compared to the other groups. However, this finding contradicts the study by Kokich et al.,[15] wherein orthodontists were identified as the most discerning group compared to general dentists and laypersons. It’s important to note that these observations are not directly comparable to our study, as oral and maxillofacial surgeons and predictions made by AI were not included in their investigation. Among all dental specialties, orthodontic treatment and orthognathic surgery have the most significant impact on facial appearance. Consequently, these two specialists may be more attuned to evaluating facial appearances compared to other dental professionals.
The evaluators were asked to identify the regions of the face that were nearly identical between the actual and AI-predicted images. The base of the nose and the chin emerged as the most similar regions, suggesting that the changes occurring in those areas following orthodontic treatment were insignificant. This observation can be attributed to the typical retraction of the upper and lower lips during orthodontic therapy, coupled with an increase in the nasolabial angle, particularly in bimaxillary patients undergoing first premolar extraction. Since the nasolabial angle is predominantly formed by the base of the nose and the upper lip, the observed increase in this angle is primarily due to the retraction of the upper lip rather than alterations in the base of the nose.[4] In our study, we noted a class I skeletal base among bimaxillary patients, where extraction resulted in more retraction of the lower lip and minimal changes in the soft-tissue chin. These findings may be influenced by alterations in soft tissue dynamics that counteract the effects of dental retraction. However, it is essential to acknowledge that while changes in the base of the nose and chin are more readily appreciated in the profile view, only frontal views were assessed in our study. In addition, our study focused on non-growing bimaxillary patients aged 18–30 years, which further impacts the observed changes in the nose and chin region. These findings are consistent with a study by Conley and Jernigan, where no significant changes were found in the supramentale, pogonion, or subnasale areas relative to glabella vertical, following extraction of all first bicuspids and retraction.[18] However, the upper lip-to-teeth ratio and gingival visibility were identified as the least identical regions. The limited predictability associated with the upper lip’s response to orthodontic tooth movement may stem from the complex anatomy and/or dynamics of the upper lip.[19]
On comparing the perceptions of laypersons and dentists, it became apparent that the acceptance of the AI-predicted images was higher among laypersons compared to dentists. This discrepancy can be attributed to the fact that, unlike professionals, laypeople are not accustomed to evaluating facial appearance from a scientific perspective.
Notably, the age and gender of the evaluators did not appear to influence their responses when assessing the AI-predicted and actual post-treatment images. A study by Flores-Mir et al. and Imani et al.[20] included a questionnaire that collected self-perceptions of various evaluators regarding their dentofacial esthetics and orthodontic treatment needs. The study revealed that age and gender play an insignificant role in the responses made by evaluators.[20]
To the best of our knowledge, this study was the first of its kind. Given that we were unaware of any similar study predicting the outcome of orthodontic treatment using pre-treatment frontal facial photographs of bimaxillary patients who underwent extraction; it was challenging to compare our results with those available in the literature.
Anticipating facial appearance through digital technology has become prevalent in various fields such as artistry, criminology, and plastic and cosmetic surgery.[21-23] Similarly, digitization holds the potential to revolutionize orthodontic diagnosis and treatment planning. Advances in computing power and AI were bound to have a significant impact on orthodontic specialty, despite their minor limitations.
A larger dataset is recommended for training the algorithm to achieve improved accuracy. However, the sample size in our study was limited to 50, designating it as a proof-of-concept study.
Only records of class I patients with bimaxillary protrusion who underwent extraction of all first bicuspids were included in the study. Future research should encompass all malocclusion types for comprehensive evaluation.
Unequal gender representation in the study, initially with 18 male and 32 female patients, could introduce potential biases. Although subsequent analysis involved a near-equal distribution of genders (6 females and 4 males), it is crucial to acknowledge and address potential gender bias in observers’ perceptions for future studies.
Minor variations in predicted images were observed due to differences in tonicity and proportions. Future studies should adhere to stringent standardization of pre-treatment and post-treatment photographs to mitigate such variations.
The study’s outcome was promising as the algorithm’s predictions were realistic and comparable to the actual post-treatment outcome. Future research should focus on standardizing this strategy of using AI prediction before orthodontic treatment, employing larger datasets, patients from diverse age groups, and various malocclusion types treated with different modalities.
CONCLUSION
In summary, the integration of AI in predicting the outcomes of orthodontic treatment presents promising advancements for clinical practice. Our study showcases the effectiveness of the StyleGAN-2 algorithm in accurately predicting post-treatment facial and dental outcomes. While more than 50% of evaluators found the AI predictions reliable, varying levels of accuracy were observed across different facial areas. The base of the nose and chin exhibited the highest accuracy, whereas gingival visibility and the upper lip-to-teeth relationship demonstrated the least accuracy. Challenges such as altered image tonicity and proportionality highlight the need for further refinement.
Despite these limitations, the hierarchical acceptance of AI-predicted images among evaluators suggests its potential clinical utility. Laypeople exhibited the highest acceptance, followed by other specialty dentists, orthodontists, and oral maxillofacial surgeons. These findings underscore the promising role of AI in orthodontic diagnosis and treatment planning, emphasizing the importance of future research to address limitations and standardize AI integration into orthodontic practice.
Ethical approval
The research/study approved by the Institutional Review Board at Institutional Ethical Committee – Ragas Dental College and Hospital, number 20210405, dated 24-2-2022.
Declaration of patient consent
The authors certify that they have obtained all appropriate patient consent.
Conflicts of interest
There are no conflicts of interest.
Use of artificial intelligence (AI)-assisted technology for manuscript preparation
The authors confirm that there was no use of artificial intelligence (AI)-assisted technology for assisting in the writing or editing of the manuscript and no images were manipulated using AI.
Financial support and sponsorship
Nil.
References
- Bimaxillary dentoalveolar protrusion: Traits and orthodontic correction. Angle Orthod. 2005;75:333-9.
- [Google Scholar]
- Profile changes following orthodontic treatment of bimaxillary protrusion in adults with the Begg appliance. Eur J Orthod. 1989;11:375-81.
- [CrossRef] [PubMed] [Google Scholar]
- Mandibular changes in persons with untreated and treated Class II division 1 malocclusion. Am J Orthod Dentofacial Orthop. 1998;113:661-73.
- [CrossRef] [PubMed] [Google Scholar]
- Soft tissue changes following the extraction of premolars in nongrowing patients with bimaxillary protrusion: A systematic review. Angle Orthod. 2010;80:211-6.
- [CrossRef] [PubMed] [Google Scholar]
- Prediction of lip response to four first premolar extractions in white female adolescents and adults. Angle Orthod. 2009;79:413-21.
- [CrossRef] [PubMed] [Google Scholar]
- Orthodontic incisor retraction caused changes in the soft tissue chin area: A retrospective study. BMC Oral Health. 2020;20:108.
- [CrossRef] [PubMed] [Google Scholar]
- Prediction accuracy of Dolphin software for soft-tissue profile in Class I patients undergoing fixed orthodontic treatment. J World Fed Orthod. 2022;11:29-35.
- [CrossRef] [PubMed] [Google Scholar]
- Accuracy of Dolphin visual treatment objective (VTO) prediction software on class III patients treated with maxillary advancement and mandibular setback. Prog Orthod. 2016;17:19.
- [CrossRef] [PubMed] [Google Scholar]
- Neural networks: A new technique for development of decision support systems in dentistry. J Dent. 1998;26:305-9.
- [CrossRef] [PubMed] [Google Scholar]
- Subject independent facial expression recognition with robust face detection using a convolutional neural network. Neural Netw. 2003;16:555-9.
- [CrossRef] [PubMed] [Google Scholar]
- Applying artificial intelligence to assess the impact of orthognathic treatment on facial attractiveness and estimated age. Int J Oral Maxillofac Surg. 2018;48:77-83.
- [CrossRef] [PubMed] [Google Scholar]
- Development of novel artificial intelligence systems to predict facial morphology after orthognathic surgery and orthodontic treatment in Japanese patients. Sci Rep. 2021;11:15853.
- [CrossRef] [PubMed] [Google Scholar]
- Progressive growing of Gans for improved quality, stability, and variation, [ArXiv preprint] arXiv :17.
- [CrossRef] [Google Scholar]
- A focus on reliability in developmental research through Cronbach's Alpha among medical, dental and paramedical professionals. Asian Pac J Health Sci. 2016;3:271-8.
- [CrossRef] [Google Scholar]
- Comparing the perception of dentists and lay people to altered dental esthetics. J Esthet Dent. 1999;11:311-24.
- [CrossRef] [PubMed] [Google Scholar]
- Likert scale vs visual analog scale for assessing facial pleasantness. Am J Orthod Dentofacial Orthop. 2021;160:844-52.
- [CrossRef] [PubMed] [Google Scholar]
- Facial attractiveness of cleft patients: A direct comparison between artificial-intelligence-based scoring and conventional rater groups. Eur J Orthod. 2019;41:428-33.
- [CrossRef] [PubMed] [Google Scholar]
- Soft tissue changes after upper premolar extraction in Class II camouflage therapy. Angle Orthod. 2006;76:59-65.
- [Google Scholar]
- Soft-tissue profile changes resulting from retraction of maxillary incisors. Am J Orthod Dentofacial Orthop. 1987;91:385-94.
- [CrossRef] [PubMed] [Google Scholar]
- Self-perceived orthodontic treatment need evaluated through 3 scales in a university population. J Orthod. 2004;31:329-34.
- [CrossRef] [PubMed] [Google Scholar]
- Photo-realistic face age progression/regression using a single generative adversarial network. Neurocomputing. 2019;366:295-304.
- [CrossRef] [Google Scholar]
- Turning back the clock: Artificial intelligence recognition of age reduction after face-lift surgery correlates with patient satisfaction. Plast Reconst Surg. 2021;148:45-54.
- [CrossRef] [PubMed] [Google Scholar]
- Artificial intelligence and crime: A primer for criminologists. Crime Media Cult. 2021;17:209-33.
- [CrossRef] [Google Scholar]